Last week, I came across news announcing Digital Diagnostics’ (formerly IDx) acquisition of 3Derm, two automated diagnostics startups that I have been following closely for the past few years. While they operate within different specialties – ophthalmology for the former and dermatology for the latter – they both belong to a pre-2012 group of “first movers” into healthcare AI. These startups had already made some progress, with products on the market, before deep learning came to prominence and anything “AI/ML” became a hot topic. They were able to capitalize on their existing data infrastructure to implement deep learning solutions at scale and develop clinical AI tools. In this article, I explore how this head start has helped them, how ideas for AI-centric products can be validated without AI, and how AI offerings across multiple medical specialties fit into the larger context.
A tale of two startups
First, we have the acquirer Digital Diagnostics with diagnostic products for diabetic retinopathy – a diabetes complication that damages light-sensitive tissue in the retina (the back of the eye). If left untreated, it may cause mild vision problems and blindness in extreme cases↗. Retinal imaging using a fundus camera (a low-powered microscope with a camera) and manual interpretation by an ophthalmologist is a widely accepted screening method for this disease↗. Digital Diagnostics is developing AI systems for the grading and detection of diabetic retinopathy in fundus photographs. In 2017, Digital Diagnostics ran the first clinical trial for an autonomous medical AI system↗↗, and a year later its product received FDA clearance making it the first device authorized for fundus image screening without the need for an ophthalmologist – essentially making it usable by healthcare providers who may not be involved in eye care↗.
The acquiree, 3Derm, is developing diagnostic products for skin diseases ranging from common rashes to severe infections and skin cancer. Diagnosis of these types of diseases is often carried out initially through visual inspection with potential followup biopsy and pathological examination. Given that the average wait time to see a dermatologist in the US is 28 days↗, teledermatology has grown in popularity as a cost-effective and reliable means for dermatology care delivery↗. This involves photographic imaging of suspicious skin findings for almost instantaneous interpretation by remote experts. 3Derm provides teledermatology services through skin imaging hardware and automated diagnostic products. They’ve participated in multiple pilot programs↗ and clinical efficacy studies↗↗, and earlier this year, a 3Derm product for autonomously detecting different types of skin cancer became the first AI device in dermatology to receive the FDA “breakthrough Device designation”. This designation is a fast-track regulatory pathway for devices that demonstrate more effective diagnosis for life-threatening and irreversibly debilitating diseases↗.
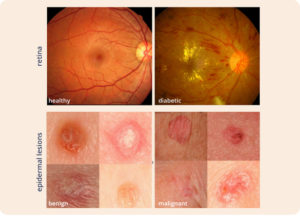
Top: Two fundus photographs. The left image is healthy while the right image shows signs of diabetic retinopathy. Bottom: Two epidermal lesion images. The left image is benign while the right image is malignant. Sources: ocutech.com & nature.com
These two startups share multiple aspects. Both are in the diagnostic space where interpretation by a specialist is required. From a machine learning perspective, both problems addressed may be formulated as a classification problem where a fundus photograph or an image of a suspicious mole can be classified into either negative or positive for a given disease. At their core, both AI applications are likely to utilize similar convolutional neural networks (CNNs) – a class of deep learning algorithms↗ – to perform this classification. Additionally, both products are primarily targeted for use in primary care where they may help triage patients and identify those who would benefit the most from a referral to specialists. Finally, the data used by both are also similar: two dimensional images captured by devices that come in handheld mobile variants and require minimal operator skill – making them ideal for non-specialist primary care settings. Perhaps the most interesting commonality between them: both were founded prior to the 2012 resurrection of research in neural networks and the popularization of deep learning. To understand how this head start has helped them capitalize on the technology, let’s explore what changed back then.